Do We Do Our Best Work for Machines?
#Technology
Casey Thompson
by
Casey Thompson
![]() |
Casey Thompson Web & Digital Media Manager |
|
|
|
Just four years ago, a study published by eSchool News predicted that AI in education and learning would increase 47.5% through 2021; as it turned out, the prediction was conservative.
The current landscape
AI and ML are being used at every step of the student and educator journey to:- Build statistical models of student knowledge, evaluating student achievement and instructor proficiency
- Streamline recruiting and reduce unconscious bias
- Create a digital “paper trail” for audit purposes
- Organize and optimize learning materials, and continually update them based on student and instructor feedback
- Create optical systems that can automatically grade students’ work with a cell phone picture
- Move toward AI-powered voice recognition systems that can help detect reading issues
- Make scheduling algorithms that can help determine optimal learning times for students and subjects
- Construct grading systems that quickly aggregate assessment data and decrease response time to student needs
- Create rule-based tutoring systems that “learn” from student errors and teacher corrections
Learn more: 6 Roles Technology Can Play In The Hiring Process
That’s all in addition to broader-scale, district-wide assessment and application.
Are the machines taking over?
To many, that sounds like technology successfully educating and preparing kids; to others, it may sound like the machines are taking over.This is particularly true when AI is used as an assessment tool, whether for employee performance or student achievement.
AI is great at aggregating data, dispassionately capturing what it “sees,” and noting what’s right and wrong when the questions are black-and-white—“What’s 17 times 27?”, for instance.
Conversely, AI struggles with assessments involving nuance, empathy, context, art, and style. A Grammarly-type grammar tool, for example, may consider a student’s non-traditional language structure to be incorrect, when it’s actually serving an artistic purpose.
In fact, the tool may consider any non-traditional use to be “wrong,” whether the reasons are artistic, cultural, contextual … or whether the usage really is wrong.
AI also struggles with intent and sentiment.
Market researchers have long wrestled with this shortcoming of AI when it’s applied to the positivity or negativity of social-media posts or focus-group transcriptions.
More recently, AI-based tools have been applied to statements made by executives on earnings calls, who often use convoluted language to make negative financial statements sound positive because they know they’re being scored on their performance.
In a school setting, that could translate into teachers “performing for the machine,” putting concepts in algorithm-friendly terms that don’t connect with students.
On the grading side, AI may consider a statement like, “I love death metal!” to be negative because it contains the word “death,” when in reality, it’s not negative at all (depending on your attitudes toward death metal).
Flagging such a statement during the grading process could actually make teachers’ jobs harder, forcing them to sift out real negativity from false.
The real value of AI
Ultimately, the real value of AI lies far from semantic analysis, and the real dangers of AI are far from that as well.AI’s value is in its potential to automate and aggregate data from the repetitive portions of grading and tutoring, while freeing up teachers and staff to interact with students on more significant levels.
AI can break education out of its “one size fits all” rut and can personalize learning to a degree that no teacher has the time or resources to replicate.
AI can even breathe new life into the staid old textbook.
The real dangers of AI
The dangers with AI lie in application design and algorithmic bias.Here’s an example of poor application design: Auto insurers often use AI-based tools that plug into a car’s onboard computer to determine whether the car’s driver is a safe driver and a low risk.
Unfortunately, the tools don’t measure safe driving but smooth driving. A driver who runs red lights in the interest of not stopping is deemed to be safer than someone who has to navigate aggressive stop-and-go traffic while being surrounded by unsafe drivers.
AI tools need to actually measure what they’re supposed to be measuring. Teacher success does not always equate with teacher efficiency.
Because of that, it’s best to combine AI evaluation tools with human supervision, at least until the measuring sticks are calibrated.
As for algorithmic bias, this cuts two ways. The most pernicious examples are when algorithms or aggregated data exacerbate divisions and discrimination.
A perfect example is Facebook’s formula for serving up ads based on race, which actually kept some people of color from seeing homes for sale in their area, even if they were home-shopping.
As an article in The Atlantic put it, “algorithms can privilege or discriminate without their creators designing them to do so, or even being aware of it.” Applied indiscriminately in schools, bad algorithms could be disastrous.
However, Big Data learnings can also help point out instances of bias, such as in a recent study that found books that won general children’s book awards, like the Newberry Award, generally showed lighter-skinned people of color than books which won identity-based awards.
For educators and administrators, AI needs to be seen as a powerful tool but still a tool—not a substitute for a human teacher or administrator. Humans still need to be in charge of the education of younger humans; AI needs to be the helpmate.
![]() |
Casey Thompson Web & Digital Media Manager |
|
|
|
|

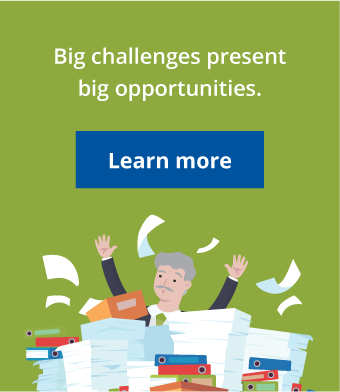